Policy Brief
An Autonomy-Based Classification
AI Agents, Liability and Lessons from the Automated Vehicles Act
Authors
Programmes
Published by
Interface
April 02, 2025
Note: This policy briefing is based on a peer-reviewed workshop paper at the 2024 NeurIPS conference (Regulatable ML, Socially Responsible Language Modelling Research, Towards Safe & Trustworthy Agents). The original paper can be found here.
in Cooperation with the Ada Lovelace Institute
Executive Summary
2025 has been proclaimed the "year of AI agents" 1 . Unlike chatbots confined to a text-based interface, AI agents can autonomously perform complex, open-ended tasks across multiple applications—ranging from scheduling meetings and ordering groceries to managing workflows and coordinating warehouse logistics. Although this promises significant efficiency gains, it also poses a central legal question: who is liable if an AI agent causes harm?
Existing legal frameworks, notably tort law, already provide foundational principles for addressing harm. However, it is yet to be seen how these doctrines will be applied to autonomous AI agents given their unique challenges, for instance for:
-
Harm Identification: Clearly identifying and proving specific harms can be challenging, particularly when harms are immaterial (such as rights violations) or systemic and observable only over time or at scale.
-
Responsibility Allocation: Determining accountability is complicated by multiple actors involved in the development, integration, and deployment of AI agents, resulting in intricate value chains and the "many hands" problem.
-
Harm Prevention: Demonstrating that harm was preventable is difficult due to the inherent unpredictability of AI agents' autonomous decision-making and potential misalignment between user intentions and agent actions.
However, this is not the first time lawmakers have grappled with autonomous technology and challenges this might pose. The UK's approach to autonomous vehicles under the Automated Vehicles Act provides a valuable parallel. This framework shifts liability from the human occupant to manufacturers or software developers once vehicles attain certain autonomy levels. Similarly, when AI agents achieve higher autonomy—significantly limiting user control—responsibility should shift to developers and upstream actors best positioned to prevent harm.
Drawing on this analogy, we propose a five-level autonomy-based classification for AI agents:
-
Lower Levels (1–2): AI agents perform narrowly defined tasks with substantial user oversight; liability largely remains with the user.
-
Intermediate Levels (3–4): Responsibility begins transitioning towards developers and integrators who enable the agent’s advanced decision-making capabilities.
-
Highest Level (5): AI agents independently decide and execute tasks with minimal human intervention; developers and providers bear greater liability due to reduced user control.
Using this taxonomy as an analytical lens offers several advantages:
-
Clearer Standards of Care: By mapping the scope of control, courts can better determine whether actors exercised reasonable care relative to their ability to prevent harm.
-
Technical Innovation Incentives: By clarifying liability expectations, this approach encourages developers to build more robust control mechanisms, such as real-time monitoring dashboards, approval workflows for high-risk actions, audit trails of decision processes, and emergency override capabilities.
Importantly, we do not need to wait until liability questions arise to begin leveraging this framework. Policymakers, developers, and researchers can proactively explore actionable steps today, such as:
-
Transparency Requirements: Implement mandatory logging of AI agent decisions and clear indicators of agent-driven actions.
-
Insurance Mechanisms: Develop specialized insurance schemes that promptly compensate victims, with insurers subsequently claiming reimbursement from responsible parties, similar to automated vehicle models.
-
Duty of Care Standards: Establish explicit, autonomy-specific guidelines outlining reasonable care expectations for users (e.g., configuration responsibilities) and developers (e.g., safeguards preventing misuse).
Ultimately, AI agents promise transformative benefits yet simultaneously blur traditional accountability frameworks. Adopting an autonomy-based classification, we suggest, is a useful first step toward developing legal categories for AI agents and establishing appropriate liability frameworks. However, realizing this vision will require collaborative efforts among policymakers, insurers, courts, and developers to effectively balance innovation with robust governance, ensuring accountability evolves alongside technological advancements.
Introduction
AI agents—autonomous systems capable of executing complex, open-ended tasks with limited human oversight—have attracted growing interest and investment in research, 2 , 3 industry 4 , 5 , 6 and policy. 7 8 9 Early examples of AI agents, such as OpenAI’s Operator, Cognition’s Devin, MultiOn’s Agent Q, and Sakana’s AI Scientist exhibit some, albeit limited degree of autonomy, enabling them to independently perform a variety of activities in domains including software engineering, online retail, and scientific research. As AI agent technology matures, its economic potential will likely grow, enabling agents to handle more diverse tasks with greater reliability and autonomy.
However, as AI systems grow more autonomous, determining who should bear liability for any resulting harm becomes increasingly complex. While existing legal frameworks in the EU (e.g., the Product Liability Directive) and the United States (e.g., tort doctrines 10 ) will probably apply to AI agents, it can be difficult to pinpoint whether developers or end users—or anyone at all—should be held accountable in cases where neither party intended or reasonably foresaw the harm. Traditional standards that hinge on “reasonable care” have yet to be clearly adapted for AI agents, making it difficult to assign responsibility among multiple contributors. This legal ambiguity introduces significant uncertainty for all involved, from developers and the broader innovation ecosystem to users and other potential affected parties, raising questions about how to handle AI-driven harms.
One area where lawmakers have grappled with comparable policy challenges is liability for autonomous vehicles (AVs). In particular, we seek to draw lessons from the UK’s approach to AV regulation, which established different levels of autonomy to guide the allocation of liability. Through the Automated Vehicle Act 2024, 11 the "user-in-charge" will not be held (criminally) liable for damages caused by the AV when it is in self-driving mode. Instead, the manufacturer or software developer are directly liable for offences resulting from ‘the way the vehicle drives’. The Automated and Electric Vehicles Act 2018 complements this by protecting AV users from civil liability claims, recognizing that when a user has no effective control over the vehicle’s operation, they should be shielded from fault. Put simply, as vehicles become more autonomous, the law increasingly shifts responsibility away from individual drivers and toward manufacturers and software developers.
Building on this analogy, our paper proposes a taxonomy for AI agent autonomy to guide courts, insurers, and other actors in assessing responsibility.
Much like AV law, categorizing AI agents by level of autonomy offers a structured lens for analyzing how existing tort doctrines might adapt to distribute liability more consistently.
It may also assist in shaping risk-based regulations on AI agents and incentivizing the development of robust control mechanisms for AI agents.
This paper proceeds as follows. Section 2 defines the key concepts underlying AI agents and tort liability. Section 3 examines the core challenges AI agents pose for allocating liability. Section 4 analyzes the UK’s regulatory approach to AVs and its implications for AI agent liability. Section 5 advances an autonomy-based taxonomy for AI agents, and Section 6 concludes with recommendations for future research.
Background
What are AI agents?
Broadly speaking, AI agents can be defined 12 as systems that can independently plan and carry out a sequence of actions on behalf of users, without necessitating continuous human supervision. They are often characterised by their ability to perceive and operate in complex environments across a variety of domains, to adapt their strategies and actions based on new input autonomously, and to interact with their surroundings, for instance, through natural language interfaces. 13 , 14 , 15 , 16
Recently, AI agents built on large-scale foundation models have garnered widespread attention, not least for their potential to handle a broad range of tasks. Unlike chatbots, these agents typically integrate “scaffolding” software—an intermediary layer that enables them to interface with external tools and environments, coordinating actions like web browsing, code generation, or data retrieval. Nevertheless, considerable uncertainty remains about how these agents will evolve, including the architectures and deployment infrastructures they will adopt, and how current open challenges—such as task execution reliability and effective control mechanisms—will ultimately be resolved.
AI agents are different from earlier AI technologies in a few ways. Compared to earlier virtual assistants (e.g., Siri/Alexa), they are able to operate in the ‘real world’ with less constraints (e.g., navigate web browsers) and perform more complex and open-ended tasks. Their pathways to executing a goal are not pre-programmed, so advanced AI agents are non-deterministic. Depending on the level of ‘agenticness’ or autonomy, AI agents may also be less supervised than earlier AI applications. Additionally, AI agents may interact with other AI agents on the web, or through a multi-agent system - which may all have similarly open-ended, non-deterministic features. Web-based actions may also pose threats to transparency: it may not always be clear where, when, and by whom AI agents are deployed.
However, defining what exactly constitutes an agent is often a more complex question in practice. Previous work, 17 , 18 , 19 has argued that agent status should not be seen as binary. Rather, ’agency’, and consequently the autonomy of a system, can be defined by an interplay of various characteristics, such as
-
Goal underspecification: The ability to operate based on high-level, underspecified goals without detailed instructions. This includes functioning on open-ended tasks in the absence of constant human supervision. 20 , 21
-
Action Complexity: The scope and potential impact of actions the system can perform, encompassing tool use (e.g., web search, programming) and operation across varied environments. 22 , 23 , 24
-
Adaptability: in their approach to pursuing a goal, by not only being able to make decisions that are “temporally dependent upon one another,” 25 but also capable of behaving differently when circumstances change.
What is tort law?
Liability refers to the legal responsibility one bears for actions (or omissions) that cause harm to others, often requiring the liable party to compensate or otherwise remedy the harm. Although liability may arise under different areas of law—such as criminal, contract, or tort—this piece focuses on tort law, which enables an injured party to seek compensation even when no contract exists between the parties.
One core principle within tort law is negligence, which imposes a general duty to take “reasonable care” to avoid harming others. 26 In practice, this means a party can be held liable if they fail to act as a reasonably prudent person would under similar circumstances and thereby cause harm–even in the absence of any specific legislation imposing liability to an actor. A critical part of determining negligence is whether the resulting harm was foreseeable: if a reasonable person in similar circumstances could anticipate the type of harm that occurred, then a duty to guard against it likely arises. In evaluating whether someone acted with reasonable care, courts look to industry standards and best practices, academic research (for instance, on human-computer interaction), guidance from policymakers, and applicable legal requirements. As our understanding of a product’s risks and mitigation approaches evolves—whether through new evidence or shifting technological standards—courts and legislatures accordingly refine their view of “reasonable care,” shaping how individuals and businesses must act.
A key procedural feature of negligence law is the burden of proof: generally, the injured party (plaintiff) must show that the defendant’s lack of reasonable care caused the harm. In some high-risk or complex scenarios, however, lawmakers or courts may shift or ease this burden to ensure that harmed parties can hold responsible actors to account—even if proving fault is technically challenging. This approach also appears in AV law, as we will discuss below.
A different standard is strict liability, which usually applies to ‘abnormally dangerous activities’ and can hold actors liable even if they behaved reasonably. Under strict liability, an actor is liable for harm even in the absence of evidence that they could have prevented such harm by exercising more care. Strict liability can therefore be applicable when it is clear which actor should be responsible, but it is hard or disproportionately onerous for the affected person to prove a breach of a duty of care. In this way, actors can still be incentivized to take more care to prevent harm, or reconsider engaging in dangerous activities, in situations where evidence of their responsibility is hard to obtain.
What Challenges Do AI Agents Introduce for Liability?
As explained in the previous section, the attribution of tort liability involves three steps: first, identifying a harm; second, showing that a particular actor was responsible for that harm; and third, proving that said harm could have been prevented with reasonable care. AI agents introduce legal challenges on all three fronts – the harms resulting from their use are difficult to identify, hard to trace back to specific actors, and are not always clearly avoidable through reasonable care. Many of these challenges also hold for AI systems and even software more generally, but are significantly exacerbated with increasing levels of autonomy.
Harm Identification: Tort liability typically requires the identification of material damages.
-
Immaterial Harm: As with AI systems generally, harms resulting from AI agents may often be immaterial, like violations of fundamental rights, or may include ‘pure economic loss’ like lost potential earnings from a lost job opportunity.
-
Systemic Harm: Further, AI agents may cause systemic harms that may take longer to identify and are only observable on a larger scale (e.g., misinformation, macroeconomic impacts). At the same time, because they directly act in their environments, AI agents may sometimes cause harms that are more tangible than those resulting from non-agentic AI systems (e.g., incorrect purchases, scam calls). Some harms from AI agents might thus be more likely to be covered by liability, whereas others will be equally difficult to substantiate as those from other AI systems.
Responsibility Allocation: Harms resulting from the use of AI agents may be difficult to trace back to particular responsible actors.
-
The “many hands problem”: As with other AI systems, AI agents often have complex value chains in which different actors carry out different stages of development (e.g. data scraping and selection, foundation model training, fine-tuning, development of scaffolding software, interface design, etc.) 27 , 28 . Consequently, it may be difficult to prove that the actions (or lack thereof) of any individual actor resulted in harm, and downstream deployers often unjustly bear the brunt of the legal burden due to power disparities in contract negotiations 29 , 30 In the case of AI agents, this problem may be compounded by the presence of multi-agent systems 31 in which responsibility is further diffused through interaction between agents (including those with different developers and users), as well as delegation of tasks by AI agents to other agents or humans, resulting in an explosion of the set of actors potentially associated with a harm.
-
Invisibility: In a recent paper by Alan Chan et al., the “visibility” of AI agents is described as knowing “when, where, how, and by whom certain agents are being used.” 32 Absent appropriate safeguards, AI agents may often be invisible. Since individuals do not always know when they are interacting with an AI agent (and thus potentially being harmed by one), they may be unable to hold developers and deployers accountable.
Harm Prevention: The complexity and autonomy of AI agents makes it hard to demonstrate the preventability of harms – i.e., that a harm would not have occurred if an actor had exercised reasonable care.
-
Unpredictability: AI systems in general are non-deterministic and as such unpredictable. AI agents, by autonomously interacting with their environments, may dramatically increase this unpredictability. As a result, harms may often be unforeseeable, and therefore not obviously avoidable through greater care. Further, despite some helpful developments like chain-of-thought reasoning, the black box nature of AI agents makes it difficult to explain their (mis)behavior – and therefore, to prove a link between a lack of reasonable care and harm.
-
Misalignment with user intentions: Although even the most advanced AI agents currently on the market still require regular check-ins and sign off by users, future AI agents may require less oversight. In these scenarios, AI agents’ failure to correctly interpret user instructions may lead to unintended and unforeseen behavior. Resultant harms raise challenging questions about standards of care: should the user have exercised greater care in prompting the AI agent, or should the developer have exercised greater care in ensuring that the AI agent can interpret ambiguous instructions?
Liability & Autonomy: A Case-study in Autonomous Vehicles
In earlier sections, we noted that AI agents operating with minimal human oversight pose unique liability challenges. This section uses autonomous vehicles as a real-world example of how lawmakers address the balance between autonomy and control in liability. While AVs differ from AI agents in physical embodiment and narrower scope, the UK’s regulatory framework highlights a broader principle: as autonomy increases, the locus of control shifts away from the end user and toward the technology’s upstream developers.
Overview: An Autonomy-Focused Approach in AV Law
A defining feature of the UK’s approach to AVs is its reliance on levels of autonomy to clarify where control resides at any given time. Smply speaking, when an AV drives itself with limited or no input from the occupant, the occupant is no longer treated as the “driver” for liability purposes. Instead, responsibility attaches to upstream entities such as manufacturers or software developers, who design and maintain the autonomous functionality. In addition, insurance ensures quick compensation to victims, and insurers can subsequently pursue the parties most capable of preventing harm. .
Both the the Automated and Electric Vehicles Act 2018 (covering civil liability) and the Automated Vehicles Act 2024 (covering criminal liability) use a classification scheme based on levels of autonomy: at lower levels, the human driver retains primary control and may be liable for mistakes; once the vehicle meets or exceeds a threshold where it can drive itself independently, liability shifts away from the occupant. Full details on these classification systems—such as those from the Society of Automotive Engineers (SAE) and the Association of British Insurers (ABI)—are available in the appendix.
Key Takeaways from the UK AV Approach
In sum, the UK’s autonomy-focused approach—anchored in the Automated Vehicles Act 2024 and the Automated and Electric Vehicles Act 2018—sets a legal precedent by centering liability determinations on the level of control. The following principles, drawn from the AV context, offer some insights for AI agent liability:
-
Linking Liability to Control: When the user can no longer actively oversee critical functions (e.g., steering, braking, or decision-making), the law treats the developer or manufacturer as the responsible party. This principle can equally apply to AI agents, where “control” might involve deciding how or when the agent acts.
-
Recognizing Autonomy as a Spectrum: By classifying vehicles into different levels of autonomy, regulators can identify when control effectively transitions from the user to the technology, ultimately allowing for more nuanced assessments about who is responsible.
-
Gradual Transfer of Liability: The UK AV model also accounts for transitional phases—when control can be returned to the user given adequate time or warning. In AI agents, equivalent “handoff” points might involve prompts or override features that shift responsibility back to the user if they are able (and required) to intervene.
-
Upstream Accountability and Swift Redress thorugh insurance: Compulsory insurance ensures that the injured party is compensated promptly. Insurers then recoup damages from the party (e.g., a manufacturer or software developer) best positioned to prevent the harm. For AI agents, a comparable structure could hold foundational model providers or tool integrators accountable when the end user lacks meaningful oversight.
-
Information Sharing and Transparency: Legal obligations in the AV sphere require manufacturers to share operational data, enabling insurers, regulators, and courts to identify which actor is at fault. Similarly, AI agent liability frameworks might mandate the logging of agent actions, “agent IDs,” or other transparency mechanisms to clarify when user control is lost and who is ultimately responsible.
Collectively, these considerations illustrate a core concept: Where autonomy expands and control diminishes for the user, liability generally shifts to the entity that provides the autonomous capability.
Taxonomy: An Autonomy-Based Classification of AI Agents
Drawing on the lessons from AVs, we propose using similar autonomy levels to categorize AI agents. This taxonomy – leaning on previous work by Morris et al (2024) 40 and Mitchell et. al (2025) 41 -- recognizes that an agent's degree of autonomy directly influences the extent of control users can realistically exercise—which in turn should inform how liability is allocated. Just as the UK's Automated Vehicle Act 2024 shifts responsibility from users to developers when vehicles operate independently, a similar principle can guide liability distribution for AI agents.
Proposed Classification Framework
To clarify the relationship between autonomy and liability, our taxonomy identifies five levels of AI agent autonomy, ranging from simple (Level 1) to fully autonomous (Level 5). In each level, we consider the:
-
Generality and scope of functions: Does the agent handle narrow, predefined tasks or broader, open-ended goals? Put differently, is the agent a "General Purpose" agent?
-
Control: Who decides when the agent acts—the user or the system itself? Who determines how the agent completes a task—the user or the system?
-
Access to external tools/environments: Does the agent operate in a closed system, on limited domains, or in an open environment (e.g., full web access)?
We group actors into two broad categories, the developer and the user. On the developer side, this label collectively refers to actors who build the base model, supply the “scaffolding” infrastructure, or otherwise shape the AI agent’s functionalities. Because it is difficult for an affected party to pinpoint which upstream contributor exercised key control, an effective liability regime will often hold these developers jointly responsible (similar to grouping vehicle manufacturers and software providers in AV law), thereby easing the burden on individuals seeking redress.
Figure 2: We have updated the table in an earlier version of this paper inspired by a recent table published by hugging face, which similarly describes AI agent levels of autonomy based on the rangefunction, “when,” and “how” of functions, but leaves out the ‘access to the world’ variable and description of the user’s role at each level.
Combining these levels of autonomy with the allocation of liability in AV law can provide some rules of thumb in assigning liability across the AI agent value chain. In the UK’s Automated Vehicle Act 2024, users are liable when they are ‘in charge’ of the vehicle. Applied to AI agents, we might similarly expect liability to accrue with users who are more clearly ‘in charge’ of the agent’s actions (level 1-3 agents) but to be distributed away from the user when they have less control over the agent’s actions (level 4-5 agents).
Viewing AI agents on an autonomy spectrum helps situate AI agents within a broader context of technical development. Unlike earlier forms of agentic AI, ‘advanced’ AI agents currently in development are able to execute a larger range of functions (or even become general-purpose), can operate in an open environment, and can complete goals via non-deterministic pathways. In comparison, earlier AI agents had more basic functions, like controlling smart appliances, or automating specific tasks, and any interactions were mediated through pre-approved APIs and web domains.
Merits and Role of an AI Agent Taxonomy
A taxonomy can guide courts in understanding each actor’s standard of care by mapping out their sphere of control. Drawing on parallels with the UK’s automated vehicle framework, we argue that liability for AI agent harms should attach to those who are best placed to control how the agent operates. The accompanying table shows how agent capabilities intersect with the user’s influence—whether by choosing the agent’s domain, crafting prompts, monitoring built-in safeguards, or intervening when needed. This classification highlights differences between limited-function agents and more general-purpose ones, focusing on who decides when and how tasks are carried out, and the degree of access the agent has to external environments. Accordingly, systems handling a few narrow tasks (Levels 1–2) warrant a different standard of care than those pursuing broad, open-ended goals (Levels 4–5), whose outcomes are less predictable and harder to address. Although the taxonomy does not resolve every issue noted in Section 3, it does clarify both the extent of an AI agent’s capabilities and the levers of control available to developers and users at each autonomy level. If liability hinges on the actions of a “reasonable actor,” then understanding the scope of user intervention at each level is crucial.
In addition to helping clarify the standards of care relevant to negligence-based liability, the taxonomy may also inform policymakers in deciding when forms of strict liability may be appropriate. As stated in Section 3.2, strict liability is generally limited to dangerous activities that carry a level of risk even when appropriate care is taken. This level of risk is not likely to be present in the lower autonomy levels of AI agents (Level 1-3) due to their more limited range and scope of actions. Level 4 agents may or may not meet this threshold depending on whether built-in checks (such as limiting the domains that the agent can interact with, requiring approval for plans, and sign off for transactions) sufficiently diminish risk. Agents at Level 5 autonomy may meet this threshold of risk, as they have very open-ended access and opportunities for human oversight are more limited.
Beyond guidance for interpretation in tort low, a taxonomy may inform the application of regulations. It is not yet clear how AI Agents may be classified under existing AI regulations. In the EU, they will at least partially be addressed under the rules for GPAI models, but rapid proliferation may require agent-specific updates to the law. In other jurisdictions which consider regulation, such as the UK (which is expected to propose a ‘frontier AI’ bill in 2025), an autonomy classification could help with designation of models and/or systems which should be in scope. As we illustrate in the appendix, traditional risk-based classification, based solely on capabilities and compute thresholds, or those focusing on use-cases might be insufficient.
Finally, distinguishing between autonomy levels might incentivize technical work on control mechanisms for AI agents. If legal standards recognized different autonomy levels for AI agents, developers would be motivated to build more robust oversight and intervention features in order to reduce liability risk. For example, if Level 5 agents—those capable of performing major tasks with minimal human input—were held to a higher standard of care, developers might favor Level 3 or 4 agents with explicit control points (such as user approvals for financial transactions). This distinction could drive industry best practices around controllability, enabling real-time intervention and reducing the likelihood of costly mistakes or misuse. By contrast, if the law failed to differentiate among autonomy levels, there would be little incentive to create tools or protocols that give users meaningful control over highly autonomous systems, and progress on safer and more accountable AI could stall.
Limitations
We recognise that our analysis for AVs does not map perfectly onto all AI agents: AVs pose an obvious and direct risk to life and, although they also operate in a complex environment requiring complex decisions, they operate in a somewhat bounded domain. Moreover, as the UK 2024 AV Act was passed just last year and AVs are not actually used on British roads yet, there is little empirical evidence to support the effectiveness of the liability regime created by the Act in creating desirable liability incentives, providing protection to drivers from undue liability burdens, and ensuring quick redress for affected third parties. Still, the Act is a good example of preemptive regulation that was passed to create conditions for safe future use of a new technology and create conditions of trust for users and the general public.
Also, crucially, the UK 2024 AV Act introduces an authorisation regime, i.e., it requires AVs to be authorised before they are deployed on British roads. A comparable authorisation regime for AI agents does not yet exist, meaning that there is no similar ‘seal of approval’ from authorities that provides an assurance of safety to users. This means that there is a larger role for the user in taking reasonable care when deciding to use an AI agent and their selection of a specific agent to use, and a bigger role for the developers in providing clear information and documentation about the capabilities and safety of their AI agent.
Emphasizing users’ duty of care to make informed deployment decisions is necessary to avoid moral hazard: especially at the highest level of autonomy (level 5), a user may have less opportunity to exercise control over an agent, which would mean that the user is largely shielded from civil liability if the principle ‘less control should lead to less liability’ is applied uncritically. However, this might lead to users carelessly deploying very autonomous AI agents in the belief that they will be ‘off the hook’ for any damage the agent causes, which would be undesirable. An optimal distribution of liability will leave neither party fully off the hook: users should be incentivized to take care in choosing to deploy an agent and overseeing it;, and developers should be incentivized to develop and deploy safety practices, share information about agent performance and safety, build in sufficient opportunities for human oversight in line with reasonable expectations, and include safeguards for where such oversight might fall short (for instance based on human-computer interaction research).
Further, a key challenge for using such a framework to investigate liability is operationalizing the different levels of agent autonomy.
42
Developing ecologically valid benchmarks for agent autonomy remains an open research question,
43
,
44
as it requires consideration of not only capabilities across a wide range of tasks, but also agent affordances (e.g., tools, deployment constraints)
45
and human-AI interaction paradigms.
46
,
47
Finally, one commonly voiced concern about AV liability is that heightened standards may stifle innovation, as manufacturers wary of legal exposure could scale back new features 48 . Although conclusive empirical evidence on this remains limited, some scholars argue that stricter liability could deter smaller or less-resourced firms, also delaying potential societal benefits of new agents. At the same time, the introduction of liability might incentivise more research and innovation on safety features that ultimately would allow for safer deployment.
Outlook and Suggestions for Further Research
The increasing autonomy of AI agents poses distinct challenges for tort law and liability frameworks. Complex value chains, principal-agent relationships, and technical opacity make it difficult to identify responsible parties when AI agent actions result in harm. Drawing inspiration from automated vehicle taxonomies and regulation, we have proposed an autonomy-based classification for AI agents that can inform the development of appropriate standards of care across the AI agent value chain. Our framework supports a gradual reduction (though not complete elimination) of end-user liability when users cannot reasonably exercise effective control over an agent's actions—a principle reflected in the UK's Automated Vehicles Act 2024.
However, fully operationalizing this framework, and defining a standard of care, requires further interdisciplinary research. This could, for instance, be partially informed by how control is determined in AV law. For example:
-
How can we define that a user is ‘in charge’ of an agent, or that they are in a position to ‘exercise control’?
-
What is the range of actions that users have at their disposal for exerting control over an agent?
-
In AV law, users are not liable when they cannot control ‘steering, accelerating, or breaking’. Can we define similar parameters to determine when a user is in control for AI agents?
-
Some AVs alert a human driver to take over control in certain situations: what should the equivalent be for AI agents?
-
Some AVs, as well as their ’user-in-charge’ features, need to be ’authorised’ by the regulator. Should Level 5 autonomy need authorisation for use in certain high-risk (or open ended) contexts?
When seeking to answer these questions, developers and application providers should always use the test of how a ’reasonable average person’ would interact with the AI system. Such research can help define a standard of care for human control and subsequently, allocation of liability for AI agents.
Beyond conceptual questions, there are actionable measures that researchers, developers, and policymakers can implement immediately to improve transparency, strengthen oversight, and clarify liability. Such initiatives might include:
-
Logging and Monitoring: Establishing systems that record agent decisions, handoffs, and operating data, making harmful outcomes easier to analyze and address.
-
Documentation and Disclosure: Requiring standardized documents that outline an agent’s capabilities, intended uses, and safety protocols, so stakeholders understand operational boundaries.
-
Risk Assessments and Evaluations: Mandating formal audits and testing for higher-autonomy agents to confirm baseline safety and performance before deployment.
-
Identification Protocols: Labeling when AI agents—rather than humans—are responsible for a task, enabling clearer attribution of outcomes.
-
Certification and Registration Systems: Creating registries or approval processes for advanced-autonomy agents, much like existing regulatory frameworks for complex technologies.
Answering these technical and policy questions will require close collaboration across disciplines. Legal frameworks must adapt to rapidly evolving AI capabilities, while technological solutions should be designed with liability considerations in mind. We hope that our autonomy-based classification is a step toward aligning legal and technical domains, yet much work remains to ensure governance structures promote innovation without sacrificing accountability. Ultimately, tort law already covers AI in principle; the real question is how courts will interpret and enforce these rules in practice. Ignoring these issues will not eliminate the legal stakes—and sooner or later, they will demand clear and collective responses.
Appendix
Acknowledgements
We would like to extend special gratitude to Noam Kolt, Weiwei Pan and Siddarth Swaroop for guiding the paper’s direction and offering critical input. Special thanks to Oliver Sussman and Luisa Seeling for editing and Alina Siebert for her brilliant graphic design and publishing expertise.
We thank the organizers and reviewers of the NeurIPS Regulatable ML, Agent, and SoLaR workshops for providing a valuable interdisciplinary forum to discuss these ideas.
Further, we thank the following individuals for their helpful conversations, comments, and feedback on drafts of this policy briefing:
-
Noam Kolt (Hebrew University)
-
Afek Shamir (Pour Demain)
-
Markus Anderljung (Centre for the Governance of AI)
-
Alan Chan (Centre for the Governance of AI)
-
Peter Wills (Centre for the Governance of AI)
-
Nicole Lemke (interface)
-
Peter Cihon (US AISI)
-
Sumaya Nur (UK DSIT, Oxford AI Governance Initiative)
-
Patricia Paskov (RAND)
-
Michael Birtwistle (Ada Lovelace)
-
Andrew Strait (Ada Lovelace, UK AISI)
-
Peter Cihon (US AISI)
-
Participants of the NeurIPS Regulatable ML Workshop
-
Participants of the NeurIPS Agent Workshop
-
Participants NeurIPS SoLaR Workshop
Types of liability
Type of liability |
Components |
Control |
Example |
---|---|---|---|
Fault-based (negligence) |
|
Duty of care to prevent harms that are reasonably foreseeable, i.e. tortfeasor could and should have known that the harm could materialise and should have taken reasonable precautions. It was within his control to prevent the harm from happening and failed to do so. |
Cafe owner leaves open a cellar hatch whilst restocking and a customer accidentally falls into it and injures themselves; owner should have foreseen this created a risk and taken reasonable precaution (close the hatch). |
Strict liability |
|
Control plays a less obvious role: liability is assigned based on law or statute (regardless of fault/reasonable precautions taken), usually for a dangerous object or activity. |
Dog bites a person, owner took all reasonable precautions, but is still held liable if victim proves injury and that this has been caused by the dog.
An accident happens at a chemical plant causing physical injury through chemical exposure in surrounding villages. Despite the plant having taken all necessary precautions and adhering to industry safety standards, it The plant is automatically held liable for all physical injury damages caused by the chemical exposure. |
Vicarious liability (agency law) |
|
The principal needs to have effective control over the conduct of the agent, meaning that he could (and should) have the ability to meaningfully impact how the agent conducts their work. |
An electrician wires something in a faulty way and causes a fire, the company they work for is held liable by the homeowners for property damage. |
Product liability |
|
The manufacturer is liable for defects that occurred when the product was within his control. |
A portable charger catches on fire during normal use and causes damage. The manufacturer is held liable (unless the manufacturer can prove the charger was not defective when it left the manufacturer’s control). |
Taxonomy of Automated Vehicles
Both the Society of Automotive Engineers (SAE) and Association of British Insurers (ABI) have established taxonomies for levels of driving automation for self-driving cars.
Alternative Classification Approaches
Background: Other classification approaches
Current regulatory proposals—such as the EU AI Act and the (now-revoked) U.S. Executive Order on AI—often employ a risk-based approach
49
50
. The basic logic is that the higher the potential harm posed by a system, the more stringent its regulatory oversight and obligations should be. However, accurately quantifying such potential harm is notoriously difficult, so regulators frequently resort to simpler proxies. In practice, two main methods have emerged for gauging AI risk:
-
Capability-Based Classification: The EU AI Act’s treatment of general-purpose AI with “systemic risks” typifies a capability-centric approach, that can be e.g., measured through benchmarks or training compute 51 . Although approximating risk through an AI system’s capability levels can indicate its potential for harm, it does not fully capture how those capabilities operate in real-world settings—namely, how users interact with the environment and how much control they retain. In other words, capabilities enable certain actions but do not by themselves define risk, which ultimately depends on the deployment environment, human-AI interaction model, and end-user control 52
-
Use Case–Specific Rules: Under the EU AI Act and the proposed Liability Directive, certain applications (e.g., hiring or law enforcement) are classified as “high risk,” which entails stricter legal obligations and a potential shift of the burden of proof to the developer. While this approach works for systems confined to a single domain, it becomes more complex for general-purpose AI, which can operate across multiple contexts with varying risk levels.
Given these considerations, we propose emphasizing autonomy—the extent to which an AI system can operate independently of direct human oversight—as our principal lens for liability discussions. Although this concept is more complex to measure (e.g., while current benchmarks often rely on training compute as a rough proxy for capability, autonomy additionally involves interface design, the tools available to the system, and degrees of user oversight), a focus on autonomy can better reflect how models actually function in real-world contexts. By integrating both the system’s raw capabilities and the particulars of its deployment environment—ranging from user interaction paradigms to the broader societal backdrop–this approach might allow to account for both the system's capabilities and the real-world context in which it operates.
Table of Contents
1 Axios. (2025, January 23). 2025 is the year of AI agents, OpenAI CPO says. https://www.axios.com/2025/01/23/davos-2025-ai-agents
2 Jessy Lin, Yuqing Du, Olivia Watkins, Danijar Hafner, Pieter Abbeel, Dan Klein, and Anca Dragan. Learning to model the world with language. In Forty-first International Conference on Machine Learning. https://proceedings.mlr.press/v235/lin24g.html
3 Ziniu Hu, Ahmet Iscen, Aashi Jain, Thomas Kipf, Yisong Yue, David A Ross, Cordelia Schmid, and Alireza Fathi. SceneCraft: An LLM agent for synthesizing 3D scenes as blender code. In Ruslan Salakhutdinov, Zico Kolter, Katherine Heller, Adrian Weller, Nuria Oliver, Jonathan Scarlett, and Felix Berkenkamp, editors, Proceedings of the 41st International Conference on Machine Learning, volume 235 of Proceedings of Machine Learning Research, pages 19252–19282. PMLR, 21–27 Jul 2024. URL https://proceedings.mlr.press/v235/hu24g.html
4 Hayden Field. Ai agents are having a ’ChatGPT moment’ as investors look for what’s next after chatbots. CNBC, jun 2024. URL https://www.cnbc.com/2024/06/07/after-chatgpt-and-the-rise-of-chatbots-investors-pour-into-ai-agents.html
5 OpenAI. Research into Agentic AI Systems, dec 2023. URL https://openai.smapply.org/prog/agentic-ai-research-grants/ Accessed: September 6, 2024.
6 Maria Abi Raad, Arun Ahuja, Catarina Barros, Frederic Besse, Andrew Bolt, Adrian Bolton, Bethanie Brownfield, Gavin Buttimore, Max Cant, Sarah Chakera, et al. Scaling instructable agents across many simulated worlds. arXiv preprint arXiv:2404.10179, 2024. https://arxiv.org/abs/2404.10179
7 Julia Smakman. Ai assistants: Helpful or full of hype? Ada Lovelace Institute Blog, aug 2024. URL https://www.adalovelaceinstitute.org/blog/ai-assistants/. Accessed: September 9, 2024.
8 Michael K Cohen, Noam Kolt, Yoshua Bengio, Gillian K Hadfield, and Stuart Russell. Regulating advanced artificial agents. Science, 384(6691):36–38, 2024. https://www.science.org/doi/abs/10.1126/science.adl0625?af=R
9 Alan Chan, Carson Ezell, Max Kaufmann, Kevin Wei, Lewis Hammond, Herbie Bradley, Emma Bluemke, Nitarshan Rajkumar, David Krueger, Noam Kolt, et al. Visibility into ai agents. In The 2024 ACM Conference on Fairness, Accountability, and Transparency, pages 958–973, 2024. https://dl.acm.org/doi/10.1145/3630106.3658948
10 While, in the US, While software has traditionally been shielded from tort liability in the US, AI agents could face liability due to their potential to cause tangible real-world harms, such as financial damages through automated decision-making or physical injury through control of physical systems.
11 UK Government. Automated vehicles act 2024, 2024. URL https://www.legislation.gov.uk/ukpga/2024/10/contents Accessed: September 10, 2024.
12 For a more detailed, interdisciplinary discussion on the definition of agents, see Chopra & White p 5-27 https://press.umich.edu/Books/A/A-Legal-Theory-for-Autonomous-Artificial-Agents2
13 Stuart J Russell and Peter Norvig. Artificial intelligence: a modern approach. Pearson, 2016. http://repo.darmajaya.ac.id/5272/1/Artificial%20Intelligence-A%20Modern%20Approach%20%283rd%20Edition%29%20%28%20PDFDrive%20%29.pdf
14 Qiuyuan Huang, Naoki Wake, Bidipta Sarkar, Zane Durante, Ran Gong, Rohan Taori, Yusuke Noda, Demetri Terzopoulos, Noboru Kuno, Ade Famoti, et al. Position paper: Agent ai towards a holistic intelligence. arXiv preprint arXiv:2403.00833, 2024.
15 Yonadav Shavit, Sandhini Agarwal, Miles Brundage, Steven Adler, Cullen O’Keefe, Rosie Campbell, Teddy Lee, Pamela Mishkin, Tyna Eloundou, Alan Hickey, et al. Practices for governing agentic ai systems. Research Paper, OpenAI, December, 2023. https://cdn.openai.com/papers/practices-for-governing-agentic-ai-systems.pdf
16 Iason Gabriel, Arianna Manzini, Geoff Keeling, Lisa Anne Hendricks, Verena Rieser, Hasan Iqbal, Nenad Tomašev, Ira Ktena, Zachary Kenton, Mikel Rodriguez, et al. The ethics of advanced ai assistants. arXiv preprint arXiv:2404.16244, 2024. https://arxiv.org/abs/2404.16244
17 Alan Chan, Carson Ezell, Max Kaufmann, Kevin Wei, Lewis Hammond, Herbie Bradley, Emma Bluemke, Nitarshan Rajkumar, David Krueger, Noam Kolt, et al. Visibility into ai agents. In The 2024 ACM Conference on Fairness, Accountability, and Transparency, pages 958–973, 2024. https://arxiv.org/abs/2401.13138
18 Sayash Kapoor, Benedikt Stroebl, Zachary S Siegel, Nitya Nadgir, and Arvind Narayanan. Ai agents that matter. arXiv preprint arXiv:2407.01502, 2024. https://arxiv.org/abs/2407.01502
19 Yonadav Shavit, Sandhini Agarwal, Miles Brundage, Steven Adler, Cullen O’Keefe, Rosie Campbell, Teddy Lee, Pamela Mishkin, Tyna Eloundou, Alan Hickey, et al. Practices for governing agentic ai systems. Research Paper, OpenAI, December, 2023. https://cdn.openai.com/papers/practices-for-governing-agentic-ai-systems.pdf
20 Alexander D’Amour, Katherine Heller, Dan Moldovan, Ben Adlam, Babak Alipanahi, Alex Beutel, Christina Chen, Jonathan Deaton, Jacob Eisenstein, Matthew D. Hoffman, Farhad Hormozdiari, Neil Houlsby, Shaobo Hou, Ghassen Jerfel, Alan Karthikesalingam, Mario Lucic, Yian Ma, Cory McLean, Diana Mincu, Akinori Mitani, Andrea Montanari, Zachary Nado, Vivek Natarajan, Christopher Nielson, Thomas F. Osborne, Rajiv Raman, Kim Ramasamy, Rory Sayres, Jessica Schrouff, Martin Seneviratne, Shannon Sequeira, Harini Suresh, Victor Veitch, Max Vladymyrov, Xuezhi Wang, Kellie Webster, Steve Yadlowsky, Taedong Yun, Xiaohua Zhai, and D. Sculley. Underspecification presents challenges for credibility in modern machine learning. arXiv preprint arXiv:2011.03395, 2020. doi: 10.48550/arXiv.2011.03395. URL https://arxiv.org/abs/2011.03395.
21 Alan Chan, Rebecca Salganik, Alva Markelius, Chris Pang, Nitarshan Rajkumar, Dmitrii Krasheninnikov, Lauro Langosco, Zhonghao He, Yawen Duan, Micah Carroll, et al. Harms from increasingly agentic algorithmic systems. In Proceedings of the 2023 ACM Conference on Fairness, Accountability, and Transparency, pages 651–666, 2023. https://arxiv.org/abs/2401.13138
22 Iason Gabriel, Arianna Manzini, Geoff Keeling, Lisa Anne Hendricks, Verena Rieser, Hasan Iqbal, Nenad Tomašev, Ira Ktena, Zachary Kenton, Mikel Rodriguez, et al. The ethics of advanced ai assistants. arXiv preprint arXiv:2404.16244, 2024. https://arxiv.org/abs/2404.16244
23 Sayash Kapoor, Benedikt Stroebl, Zachary S Siegel, Nitya Nadgir, and Arvind Narayanan. Ai agents that matter. arXiv preprint arXiv:2407.01502, 2024. https://arxiv.org/abs/2407.01502
24 Peter Cihon. Chilling autonomy: Policy enforcement for human oversight of ai agents. In 41st International Conference on Machine Learning, Workshop on Generative AI and Law, 2024. https://blog.genlaw.org/pdfs/genlaw_icml2024/79.pdf
25 Law Commission of England and Scottish Law Commission Wales. Automated vehicles: Joint report. https://lawcom.gov.uk/project/automated-vehicles/
26 Negligence also creates heightened duties of care where the actor has a special relationship with the harmed party or has specialized professional training
27 Anka Reuel, Lisa Soder, Ben Bucknall, and Trond Arne Undheim. Position paper: Technical research and talent is needed for effective ai governance. arXiv preprint arXiv:2406.06987, 2024.
28 Helen Nissenbaum. Accountability in a computerized society. Science and engineering ethics, 2:25–42, 1996.
29 Alexander D’Amour, Katherine Heller, Dan Moldovan, Ben Adlam, Babak Alipanahi, Alex Beutel, Christina Chen, Jonathan Deaton, Jacob Eisenstein, Matthew D. Hoffman, Farhad Hormozdiari, Neil Houlsby, Shaobo Hou, Ghassen Jerfel, Alan Karthikesalingam, Mario Lucic, Yian Ma, Cory McLean, Diana Mincu, Akinori Mitani, Andrea Montanari, Zachary Nado, Vivek Natarajan, Christopher Nielson, Thomas F. Osborne, Rajiv Raman, Kim Ramasamy, Rory Sayres, Jessica Schrouff, Martin Seneviratne, Shannon Sequeira, Harini Suresh, Victor Veitch, Max Vladymyrov, Xuezhi Wang, Kellie Webster, Steve Yadlowsky, Taedong Yun, Xiaohua Zhai, and D. Sculley. Underspecification presents challenges for credibility in modern machine learning. arXiv preprint arXiv:2011.03395, 2020. doi: 10.48550/arXiv.2011.03395. URL https://arxiv.org/abs/2011.03395.
30 Alan Chan, Carson Ezell, Max Kaufmann, Kevin Wei, Lewis Hammond, Herbie Bradley, Emma Bluemke, Nitarshan Rajkumar, David Krueger, Noam Kolt, et al. Visibility into ai agents. In The 2024 ACM Conference on Fairness, Accountability, and Transparency, pages 958–973, 2024.
31 Hammond, Lewis, et al. "Multi-agent risks from advanced ai." arXiv preprint arXiv:2502.14143 (2025) https://www.cooperativeai.com/post/new-report-multi-agent-risks-from-advanced-ai
32 Chan, Alan, et al. "Visibility into AI agents." Proceedings of the 2024 ACM Conference on Fairness, Accountability, and Transparency. 2024. https://arxiv.org/abs/2401.13138
33 James Goudkamp. Automated vehicle liability and ai. The Cambridge Handbook of Private Law and Artificial Intelligence, 2022.
34 Law Commission of England and Scottish Law Commission Wales. Automated vehicles: Joint report. https://lawcom.gov.uk/project/automated-vehicles/
35 UK Government. Explanatory notes: Automated vehicle act 2024, 2024. URL https://www.legislation.gov.uk/ukpga/2024/10/pdfs/ukpgaen_20240010_en.pdf Accessed: September 10, 2024.
36 UK Government. Automated vehicles act 2024, 2024. URL https://www.legislation.gov.uk/ukpga/2024/10/contents. Accessed: September 10, 2024.
37 Ibid.
38 Law Commission of England and Scottish Law Commission Wales. Automated vehicles: Joint report.
39 UK Government. Explanatory notes: Automated vehicle act 2024, 2024. URL https://www.legislation.gov.uk/ukpga/2024/10/pdfs/ukpgaen_20240010_en.pdf Ac cessed: September 10, 2024.
40 Morris, Meredith Ringel, et al. "Position: Levels of AGI for operationalizing progress on the path to AGI." Forty-first International Conference on Machine Learning. 2024. https://dl.acm.org/doi/10.5555/3692070.3693548
41 Mitchell, Margaret, et al. "Fully Autonomous AI Agents Should Not be Developed." arXiv preprint arXiv:2502.02649 (2025). https://arxiv.org/abs/2502.02649
42 Meredith Ringel Morris, Jascha Sohl-Dickstein, Noah Fiedel, Tris Warkentin, Allan Dafoe, Aleksandra Faust, Clement Farabet, and Shane Legg. Position: Levels of AGI for opera tionalizing progress on the path to AGI. In Ruslan Salakhutdinov, Zico Kolter, Katherine Heller, Adrian Weller, Nuria Oliver, Jonathan Scarlett, and Felix Berkenkamp, editors, Pro ceedings of the 41st International Conference on Machine Learning, volume 235 of Proceed ings of Machine Learning Research, pages 36308–36321. PMLR, 21–27 Jul 2024. URL https://proceedings.mlr.press/v235/morris24b.html
43 Anka Reuel, Lisa Soder, Ben Bucknall, and Trond Arne Undheim. Position paper: Technical research and talent is needed for effective ai governance. arXiv preprint arXiv:2406.06987, 2024. https://proceedings.mlr.press/v235/reuel24a.html
44 Anka Reuel, Ben Bucknall, Stephen Casper, Tim Fist, Lisa Soder, Onni Aarne, Lewis Hammond, Lujain Ibrahim, Alan Chan, Peter Wills, et al. Open problems in technical ai governance. arXiv preprint arXiv:2407.14981, 2024. https://arxiv.org/abs/2407.14981
45 Sayash Kapoor, Benedikt Stroebl, Zachary S Siegel, Nitya Nadgir, and Arvind Narayanan. Ai agents that matter. arXiv preprint arXiv:2407.01502, 2024. https://arxiv.org/abs/2407.01502
46 Lujain Ibrahim, Saffron Huang, Lama Ahmad, and Markus Anderljung. Beyond static ai evaluations: advancing human interaction evaluations for llm harms and risks. arXiv preprint arXiv:2405.10632, May 2024. URL http://arxiv.org/abs/2405.10632.
47 Laura Weidinger, Joslyn Barnhart, Jenny Brennan, Christina Butterfield, Susie Young, Will Hawkins, Lisa Anne Hendricks, Ramona Comanescu, Oscar Chang, and Mikel Rodriguez. Holistic safety and responsibility evaluations of advanced ai models. arXiv preprint arXiv:2404.14068, 2024. https://arxiv.org/abs/2404.14068
48 Marchant, Gary E., and Rachel A. Lindor. "The coming collision between autonomous vehicles and the liability system." Santa Clara L. Rev. 52 (2012): 1321. https://digitalcommons.law.scu.edu/lawreview/vol52/iss4/6/
49 Black, Julia, and Robert Baldwin. "Really responsive risk‐based regulation." Law and Policy 32.2 (2010): 181-213. https://onlinelibrary.wiley.com/doi/abs/10.1111/j.1467-9930.2010.00318.x
50 Hood, Christopher, Henry Rothstein, and Robert Baldwin. The government of risk: Understanding risk regulation regimes. OUP Oxford, 2001. https://academic.oup.com/book/40484
51 In particular training compute has been critised as a useful proxy (see Hooker, S. (2024) “On the limitations of compute thresholds as a governance strategy”), however a discussion of its broader usefulness is beyond the scope of this paper. https://arxiv.org/abs/2407.05694
52 Morris, M.R., Sohl-Dickstein, J., Fiedel, N., Warkentin, T., Dafoe, A., Faust, A., Farabet, C. &amp; Legg, S.. (2024). Position: Levels of AGI for Operationalizing Progress on the Path to AGI. <i>Proceedings of the 41st International Conference on Machine Learning</i>, in <i>Proceedings of Machine Learning Research</i> 235:36308-36321 Available from https://proceedings.mlr.press/v235/morris24b.html
Authors
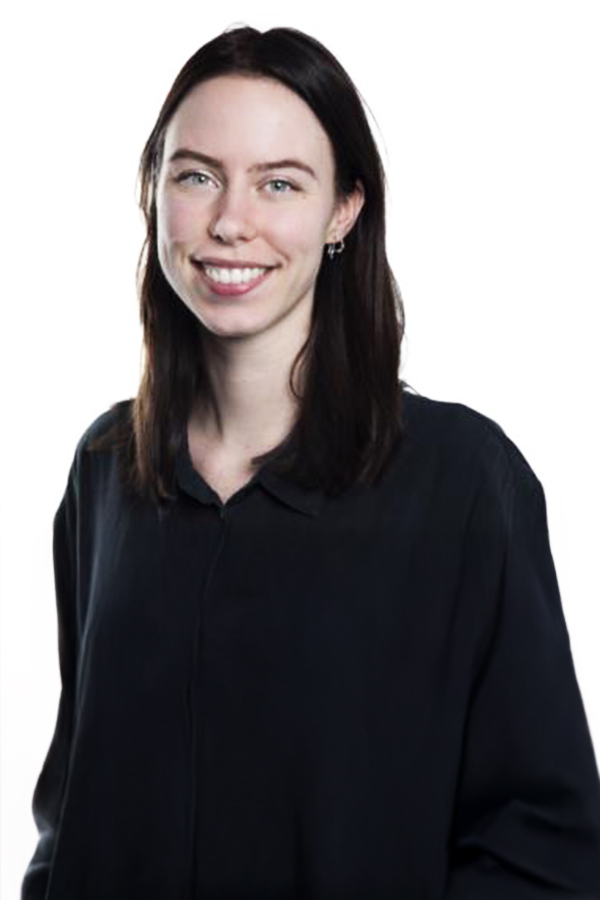
Julia Smakman
Researcher (Ada)
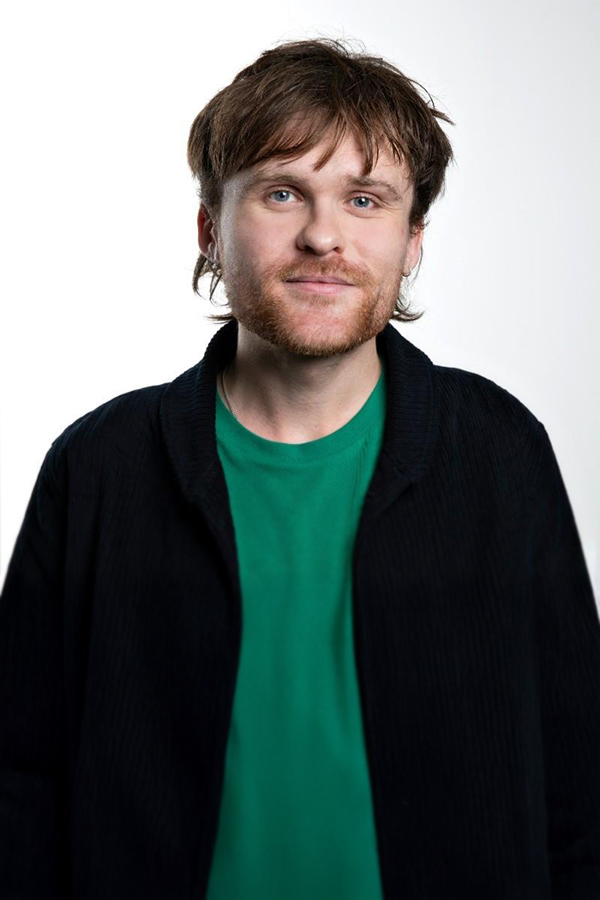
Connor Dunlop
Acting Head of EU and Global Governance (Ada)